What Happened to Big Data?
Seven years ago, I was involved in a corporate workshop headed by a customer’s CIO to discuss that company’s big data strategy. Big data was a relatively new concept back then and the workshop included four speakers who explained how it would transform that company’s business. To my surprise, not one of the speakers made a cogent point about what big data was and why the audience should care about it.One speaker talked about the “Four Vs” (I’ll let you look that up and decipher it for me because I never really understood the positioning of that definition). Another talked about open-source technologies (primarily Hadoop). Another talked about general data warehousing concepts that had been around for several years. And the fourth talked about analyzing audio files (without discussing why that was relevant for this retailer).
All the speakers talked glowingly about their topics, the exciting future, and the fact that people should sit up and take notice. People in the audience then broke into workshops to figure out how they would leverage big data. Follow up presentations discussed a myriad of analytical opportunities (some, incidentally, were about using existing techniques and technologies). At the end of the day, however, I was left with more questions about big data than when I started.
Now, several years later, after a lot of time and money has been invested, what strikes me is that I rarely hear the term “big data” anymore. So, what happened to it? In my opinion, the problem is that it’s a term that meant everything and nothing. It was a platform. It was certain data types. It was a certain set of analytical methods and tools. It was whatever you wanted it to be.
Unfortunately, what it typically didn’t have was anything to do with how the innovative use of big data and analytics would make your company better. The analytical evolution was certainly underway and creating a monumental shift in thinking, but people became fixated on a nebulous term and not pragmatic results. Whether or not people were directly involved in analytics, they owed it to themselves and their respective organizations to delve deeper into what was behind this concept and how it could be applied. Instead, a great deal of opportunity was lost chasing the hype of big data.
The Definition of Insanity
There’s an old saying, “The definition of insanity is doing the same thing over and over and expecting different results.” We see this play out time and time again in the world of analytics. Gartner has a popular concept called the “Hype Cycle.” In this cycle (see graph below) there’s an initial rush toward a new technology or concept. Expectations are inflated, which ultimately leads to a general letdown (Trough of Disillusionment) and then (hopefully) a reasonable level of common understanding whereby the technology matures and finds its stated value in the market (Plateau of Productivity).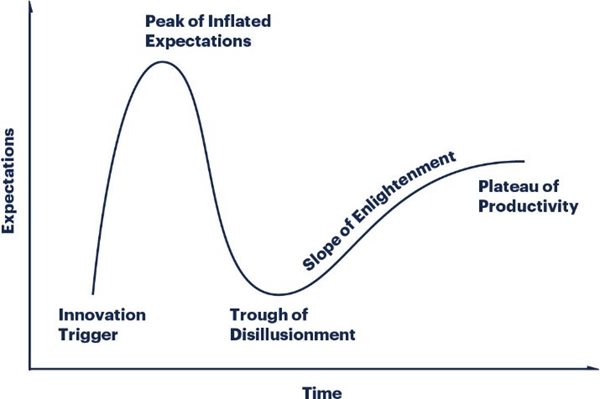
The Gartner Hype Cycle
This scenario plays out repeatedly and is particularly relevant in analytics. With respect to big data, it followed this curve but then, interestingly, dropped altogether before reaching some generally agreed upon level of acceptance. The rationale was presumably because “big data [had] become a part of many hype cycles,” according to Datanami.
The reality, in my opinion, is that the ambiguity of the term and lack of tangible value from investments ascribed to it led to its eventual downfall. The frustrating part is that this hype scenario is so prevalent that Gartner, one of the largest business and technology research organizations, identified a need for it in 1995, and its use has grown into all areas of Gartner’s consultancy scope. So, is the problem that vendors and consultants come up with terms like “big data” in the first place, or is it that we, as practitioners and enablers of analytics, keep chasing the next big thing and expect a different result? If it’s the latter, then it clearly falls into the definition of insanity.Why do we have hype cycles like those touted by Gartner? That’s a broader philosophical discussion but, on some level, it’s because of the inability to accept the responsibility of being intellectually curious when it comes to new analytical concepts (rather than taking hype at face value). Some may not be actual practitioners or developers of advanced analytics, but they still should have a base knowledge of what it is and what it’s not—and challenge partners touting these concepts to explain their relevance in a business context.
Additionally, we should focus on pragmatic aspects of the technology (will it work in my specific environment, at scale, etc.). In doing this, we’re better prepared to cut through the hype and better understand how these concepts can—or cannot—be leveraged to solve real business challenges. Note that the X axis on the graph above is “Time.” Imagine the amount of time that businesses have lost because they traversed the peaks and valleys associated with this cycle. Is it really necessary to chase windmills, or can we do better and reduce or eliminate the time lost to hype by not accepting this phenomenon as “just the way it is with analytics”?
Keeping Artificial Intelligence Out of the Hype Cycle
Today, we have a renewed focus on analytics, and the biggest term today is undoubtedly artificial intelligence (AI) and its related subsets machine learning and deep learning. Depending on who you talk to, the term AI conjures up various visions of apocalyptic movies, robots eliminating the need for humans to work, or a single algorithm you can throw a lot of data at to answer any question.None of these are realistic depictions of what current capabilities support. There are, nonetheless, a plethora of fantastic ideas about AI and what it can do, and major corporations are making huge investments based on these ideas. Unfortunately, many of these viewpoints are patently false.
Machine learning and its subset deep learning are significant innovations that opened previously unavailable opportunities in analytics. Many people, however, do not take the time to understand what the “learning” models that incorporate these methods actually do and as a result, miss the opportunity to appropriately position the techniques. Instead, they conjure up more hype around what they think it is or what someone said it is, then miss deriving real business value by making false assumptions altogether.
My latest white paper puts AI in a business context to help demystify some of the concepts. Moreover, it applies AI to a broad analytical framework to help you think about how you can use these techniques to automate/optimize an existing business process. The idea is that if you start with business challenges/opportunities, then you can better position what and how you apply analytical capabilities (including those that fall under the AI umbrella) to improve business automation and realize tangible value. At that point, it doesn’t matter what you call this or the next big idea in analytics because you’ll have what really matters—perspective.
So, don’t let what happened to big data happen to AI. Otherwise, we’ll spend our time focusing on terms and not on business solutions…at least until the next buzz phrase hits the market and we begin the hype cycle all over again, expecting (but never getting) a different result. How insane is that?!