Machine Learning Adapts to Rapidly Evolving Risk in Real-Time
Addressing the rapid evolution of fraud and risk is an imperative for payments players. Machine learning and advanced analytics can help. Find out more.
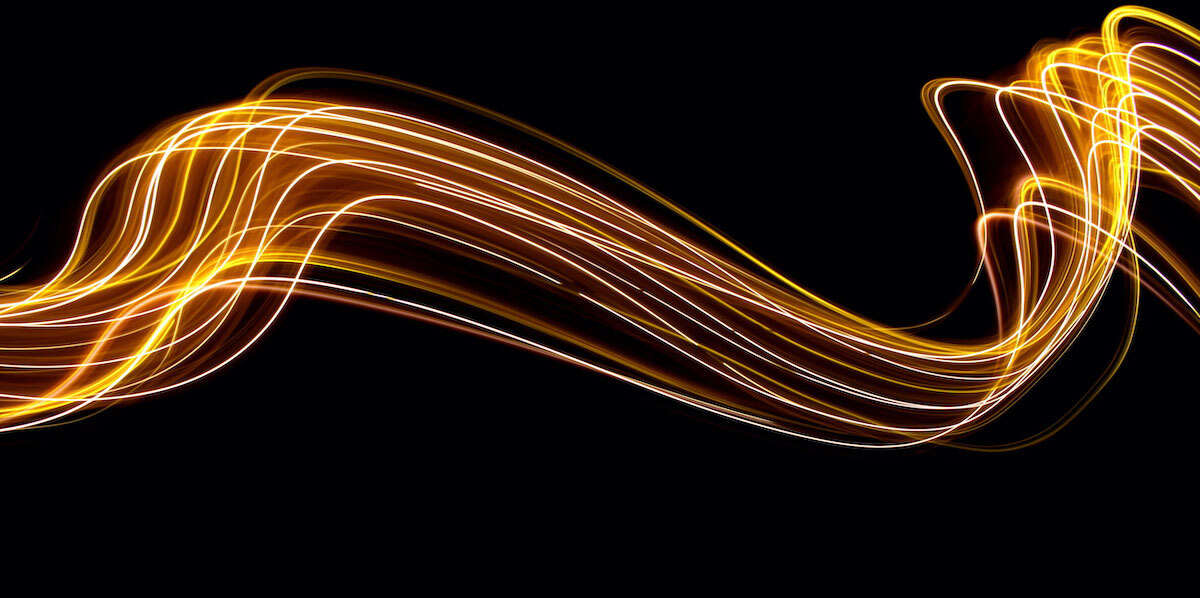
Risk is ever evolving and financial institutions must continually strive to stay ahead of that evolution by orchestrating a myriad of point solutions for fraud and credit risk. According to Shift, the U.S. suffered nearly $25 billion in payment card losses in 2018. Unfortunately, the U.S. is the most fraud-prone country in the world with more than one third of the world’s losses, growing at 15% annually. And, that fraud continuously evolves; what was once common, use of stolen card numbers gets locked down and the criminals move on to increasingly sophisticated attempts, such as account takeover.
The fastest growing type of financial crime is the ticking time bomb of synthetic identity. Synthetic identity differs from identity theft in that the entire identity, except the social security number, is fake: name, date of birth, address, etc. Criminals create thousands of these accounts, curate them for months or years to generate a high credit score, then bust out and disappear. According to The Federal Reserve’s estimates for 2016, 20% of what was attributed to credit losses was actually Synthetic Identity, costing banks $6 billion.
Synthetic identity theft is insidious and is like trying to find a single blade of grass in your lawn. Though affecting only a tiny portion of total accounts – less than one quarter of one percent – they comprise more than a quarter of overall consumer loan losses and are often miscategorized as such, rather than fraud. Banks are accustomed to ascertaining an applicant’s creditworthiness or if they are a criminal, but not accustomed to ascertaining if you’re real.
Other aspects of the financial environment are changing quickly as well, in some cases pandemic-driven. P2P scams and business email compromise are on the rise as criminals exploit every avenue to prey on peoples’ fear and uncertainty. High unemployment, economic shock and merchants in vulnerable categories result in the need to proactively anticipate delinquencies and take proactive action to optimize collections, manage credit lines and manage rising merchant risk.
Aside from anomaly detection – suspiciously thin credit files, very new social media and email accounts, bot and intrusion detection – many financial institutions are employing advanced analytics and much more data such as behavioral biometrics to authenticate customers, often eliminating the need for passwords. According to Mastercard, AI can instantly validate customer identity with over 95% accuracy. PayPal uses this type of authentication for its “One Touch” feature – running more than 1,000 checks on data such as device ID, geolocation and repeat merchant purchases – eliminating the need to log-in every time. New models can be deployed in real time, fed by a stream of disparate data types. This helps to keep PayPal a step ahead of the fraudsters.
PayPal’s approach is called “Adaptive Risk Scoring” because, unlike static rules, it adapts to the changing environment. The new EMVCo 3D Secure 2.0 uses that same approach. This tool for drastically improving the accuracy of Card Not Present authorizations for eCommerce purchases, employs hundreds of newly available transaction, consumer, authentication, merchant and device data points to yield a risk score. If the transaction seems risky or abnormal, the merchant or issuer can optionally ask for a “step-up” authentication like an out-of-band passcode sent to a mobile device. These approaches reduce false positive and negatives, according to Mastercard.
It is an increasingly dangerous and uncertain world. Newly available data elements, like autofill data, geolocation, social media account age, spend velocity, consortium data and behavioral biometrics, can be used with sophisticated analytics to instantly detect fraudulent patterns, even if they’re brand new. The analytics operate together, across ALL the data, to detect bots, account take-over, social engineering, synthetic identity and more.
.png?origin=fd)
Together, the analytics promise significant impact on business incomes includes reduction in false positives ty 20%, elimination of the needs for passwords and routing out synthetic identities. See more at “Financial Crimes Accelerator.”
Our final post in this series will explore the journey to the future of payments.
The fastest growing type of financial crime is the ticking time bomb of synthetic identity. Synthetic identity differs from identity theft in that the entire identity, except the social security number, is fake: name, date of birth, address, etc. Criminals create thousands of these accounts, curate them for months or years to generate a high credit score, then bust out and disappear. According to The Federal Reserve’s estimates for 2016, 20% of what was attributed to credit losses was actually Synthetic Identity, costing banks $6 billion.
Synthetic identity theft is insidious and is like trying to find a single blade of grass in your lawn. Though affecting only a tiny portion of total accounts – less than one quarter of one percent – they comprise more than a quarter of overall consumer loan losses and are often miscategorized as such, rather than fraud. Banks are accustomed to ascertaining an applicant’s creditworthiness or if they are a criminal, but not accustomed to ascertaining if you’re real.
Other aspects of the financial environment are changing quickly as well, in some cases pandemic-driven. P2P scams and business email compromise are on the rise as criminals exploit every avenue to prey on peoples’ fear and uncertainty. High unemployment, economic shock and merchants in vulnerable categories result in the need to proactively anticipate delinquencies and take proactive action to optimize collections, manage credit lines and manage rising merchant risk.
Aside from anomaly detection – suspiciously thin credit files, very new social media and email accounts, bot and intrusion detection – many financial institutions are employing advanced analytics and much more data such as behavioral biometrics to authenticate customers, often eliminating the need for passwords. According to Mastercard, AI can instantly validate customer identity with over 95% accuracy. PayPal uses this type of authentication for its “One Touch” feature – running more than 1,000 checks on data such as device ID, geolocation and repeat merchant purchases – eliminating the need to log-in every time. New models can be deployed in real time, fed by a stream of disparate data types. This helps to keep PayPal a step ahead of the fraudsters.
PayPal’s approach is called “Adaptive Risk Scoring” because, unlike static rules, it adapts to the changing environment. The new EMVCo 3D Secure 2.0 uses that same approach. This tool for drastically improving the accuracy of Card Not Present authorizations for eCommerce purchases, employs hundreds of newly available transaction, consumer, authentication, merchant and device data points to yield a risk score. If the transaction seems risky or abnormal, the merchant or issuer can optionally ask for a “step-up” authentication like an out-of-band passcode sent to a mobile device. These approaches reduce false positive and negatives, according to Mastercard.
It is an increasingly dangerous and uncertain world. Newly available data elements, like autofill data, geolocation, social media account age, spend velocity, consortium data and behavioral biometrics, can be used with sophisticated analytics to instantly detect fraudulent patterns, even if they’re brand new. The analytics operate together, across ALL the data, to detect bots, account take-over, social engineering, synthetic identity and more.
.png?origin=fd)
Together, the analytics promise significant impact on business incomes includes reduction in false positives ty 20%, elimination of the needs for passwords and routing out synthetic identities. See more at “Financial Crimes Accelerator.”
Our final post in this series will explore the journey to the future of payments.
最新情報をお受け取りください
メールアドレスをご登録ください。ブログの最新情報をお届けします。